Understanding the Significance of Bounding Box in Data Annotation
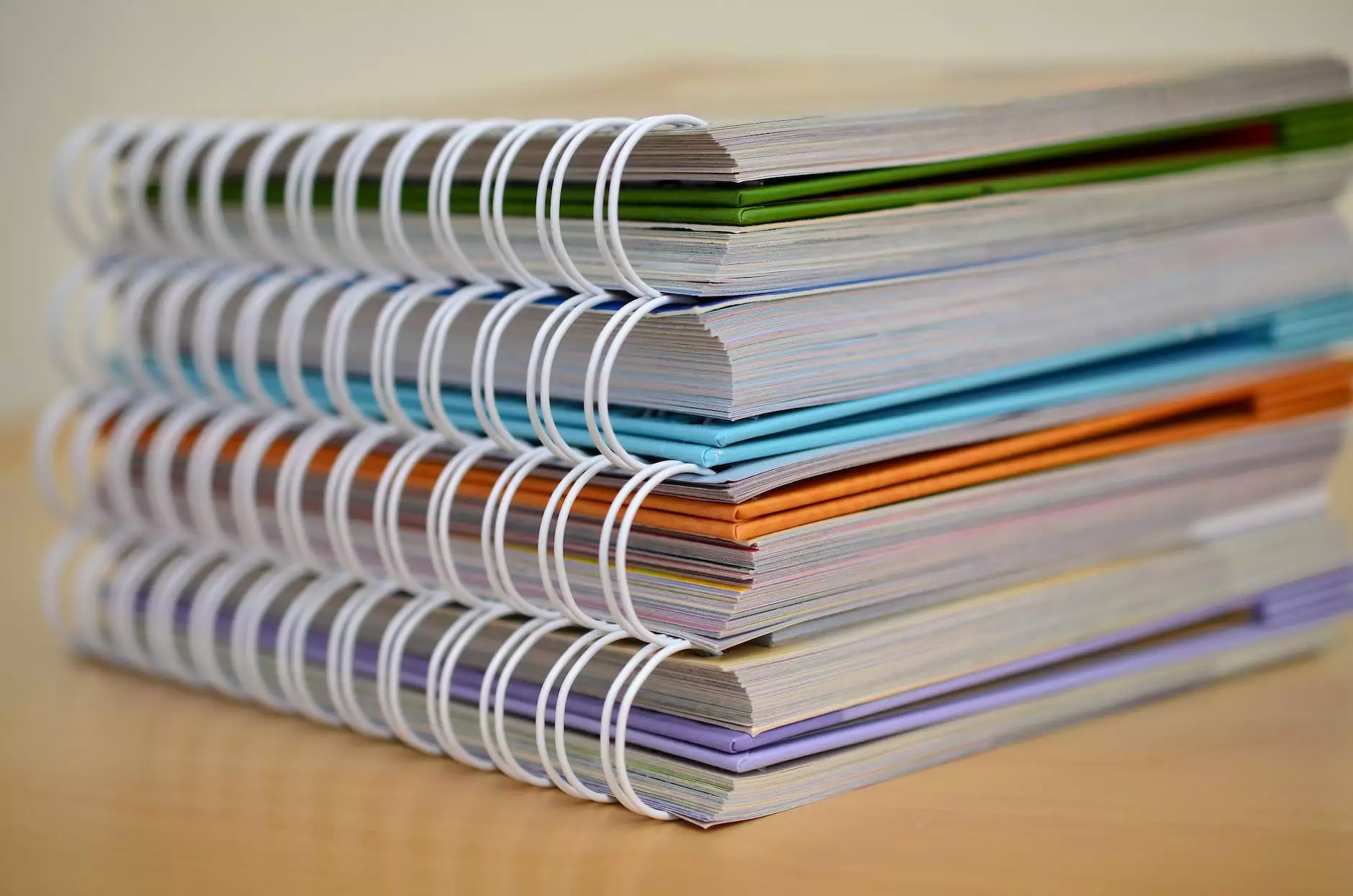
In the field of artificial intelligence, particularly in machine learning, the preprocessing of data plays a crucial role in determining the success of various algorithms. One of the most important techniques in data annotation is the concept of a bounding box. Understanding what a bounding box is, how it is applied, and its significance in environments such as KeyLabs AI can set the foundation for better machine learning outcomes. This article will delve deeply into the subject, providing a comprehensive overview that every business should embrace.
What is a Bounding Box?
A bounding box is a rectangular box that encloses an object in an image. It is defined by four coordinates: the top-left corner (x, y) and the bottom-right corner (x, y). This simple yet powerful concept forms the basis for the annotation of images, especially in tasks related to object detection.
In practical terms, think of a bounding box as a way to create a virtual frame around a specific object of interest within a visual field. For instance, if you are training an AI model to recognize cars in traffic images, each car in the image would be enclosed within its own bounding box to help the model learn the visual features associated with that object type.
The Importance of Bounding Boxes in Data Annotation
Facilitating Object Detection
One of the principal applications of bounding boxes is in object detection. This is a critical task for numerous applications such as autonomous driving, surveillance, and image search engines. By using bounding boxes, machine learning algorithms can quickly distinguish and categorize different objects in visual data.
Enhancing Model Accuracy and Performance
The precision of the bounding boxes directly impacts the accuracy of the machine learning model. Poorly defined bounding boxes can lead to misclassification and lower the model's performance. Thus, using high-quality annotations through bounding boxes ensures that the model can learn effectively and generalize well to unseen data.
Data Preparation for Training
Data preparation is essential for the success of any machine learning initiative. Bounding boxes aid in this process by providing clear visual cues that inform the model about the spatial properties and relationships of objects within an image. This aspect is especially crucial when preparing datasets for sophisticated projects like computer vision systems.
How to Create Effective Bounding Boxes
Creating effective bounding boxes is not merely a mechanical process; it’s an art that requires precision and attention to detail. Here’s how to do it right:
1. Utilize High-Quality Images
Start with high-resolution images to ensure that all objects are clearly visible. This allows for more accurate annotation and reduces the chances of overlooking details.
2. Define Boundaries Clearly
When defining the boundaries of an object, make sure that the bounding box encapsulates the entire object without cutting it off. It should touch the edges of the object without including unnecessary background space.
3. Keep Consistency
Consistency is key in data annotation. Ensure that all annotators follow the same guidelines when creating bounding boxes. This helps reduce variance in annotations and results in a reliable dataset.
The Role of KeyLabs AI in Bounding Box Annotation
KeyLabs AI offers advanced data annotation tools and platforms designed to optimize the creation and management of bounding boxes. Here are some features that set KeyLabs apart:
1. Intuitive Interface
The tools available through KeyLabs AI feature an intuitive interface that enables users to create and adjust bounding boxes effortlessly. This user-friendly design speeds up the annotation process while maintaining accuracy.
2. Automation and AI Assistance
KeyLabs incorporates machine learning algorithms to assist in the annotation process. Their tools can suggest bounding boxes based on prior models, significantly reducing the time required for manual annotations.
3. Quality Control Mechanisms
Quality assurance is a vital aspect of data annotation. KeyLabs AI implements strict quality control measures to ensure that every bounding box created is accurate and meets project specifications. Regular audits and feedback mechanisms help maintain high standards in data quality.
Best Practices for Bounding Box Annotation
1. Regular Training for Annotators
Regular training sessions for annotators help them stay updated on the best practices and techniques for creating bounding boxes. Continuous learning results in better data quality and efficiency.
2. Use of Annotation Guidelines
Develop and provide clear annotation guidelines that detail how to create bounding boxes. This includes examples, edge cases, and frequently asked questions to ensure that everyone is on the same page.
3. Iterative Review and Feedback
Utilize an iterative review process where annotations can be checked and corrected as necessary. This helps to catch errors early and improves the overall dataset quality.
Challenges Associated with Bounding Box Annotation
While bounding boxes are an effective method for data annotation, they are not without challenges:
- Ambiguity of Object Boundaries: Objects may overlap or be obscured, making it difficult to define clear boundaries.
- Varying Object Scales: Different objects may appear in varied scales due to perspective, affecting annotation accuracy.
- Inconsistent Annotation Practices: Different annotators may have varying interpretations of how to draw a bounding box, leading to inconsistencies in the data.
Future of Bounding Box Annotation
As artificial intelligence continues to evolve, so too will the methodologies surrounding data annotation. The future of bounding box annotation includes:
- Enhanced Automation: Greater integration of AI tools will aid in automating the bounding box creation process with higher accuracy, decreasing the manual workload.
- Incorporation of 3D Bounding Boxes: As technology progresses, incorporating 3D bounding boxes will become vital for applications in virtual reality (VR) and augmented reality (AR).
- Adaptive Learning Systems: Systems that learn from the feedback of prior annotations will become more prevalent, significantly enhancing their accuracy and efficiency.
Conclusion
The role of bounding boxes in data annotation cannot be overstated. They serve as the backbone of numerous machine learning applications, guiding models to make accurate predictions based on visual data. By leveraging advanced data annotation platforms such as KeyLabs AI, businesses can ensure the quality and consistency of their datasets, ultimately achieving better outcomes in their AI initiatives. Keep your organization at the forefront of technology by embracing effective bounding box techniques in your data annotation processes.